
How Algorithms Can Impact Your Net Worth
One of the most troubling trend narratives on the rise today is that of the direction intersection of tech algorithms and machine learning with that of housing and credit checks. Whether we use our residences as a much-needed retreat after work or that which is leveraged for both home and work, this area is critical because it provides our very foundation, literally and figuratively. Concerns around housing, whether redlining or other barriers, have always been issues in this country, however, the volume on the topic is growing because we are relying more and more emerging technology such as artificial intelligence to make very final decisions on crucial life plays. Such usage is important to examine because technology never exists in vacuum. Tech intersects with cultural trends, various business agendas, subconscious and conscious cultural bias, therefore, it can and is taking harmful shape across a growing number of demographics. Much of the usage of such tech is still not yet standardized nor truly discussed and analyzed as it pertains to overall human impact particularly when it comes to housing. And it's all now about to take an even more bizarre turn.
Somehow we are moving further and further from the capability of securing that portion of the American Dream which includes the house surrounded by the proverbial white picket fence to that of either an ephemeral sand castle or haunted nightmare for many. While the mortgage crisis back in 2008 completely upended many individuals and families, the next threat looms large from unrestrained, advanced algorithms that are moving like cancers on various levels.
First, there is the area of what is referred to as the affordable housing level. Evaporating at an alarming pace, this area of housing has become so critical that several of the U.S. presidential candidates are focusing on this topic as part of their overall platforms.
Former Secretary of Housing and Urban Development Julian Castro and Senator Elizabeth Warren (D-MA) are champions of an increase of affordable housing yet an increase such buildings makes nearly no difference in most cities if one has a prior eviction on his or her record. And chances are, if one has had financial issues in the past, eviction could have taken place and this blemish seems to remain on an individual's record without expiration.
Senator Cory Booker (D-NJ) seems to be the only candidate out front to call for an end to the eviction blacklisting that can easily occur via the third-party algorithmic searches that unregulated tenant credit check companies conduct at the behest of landlords without so much the batting of an eyelash and can easily prevent one from securing housing, affordable or otherwise, no matter how much of it is made available.
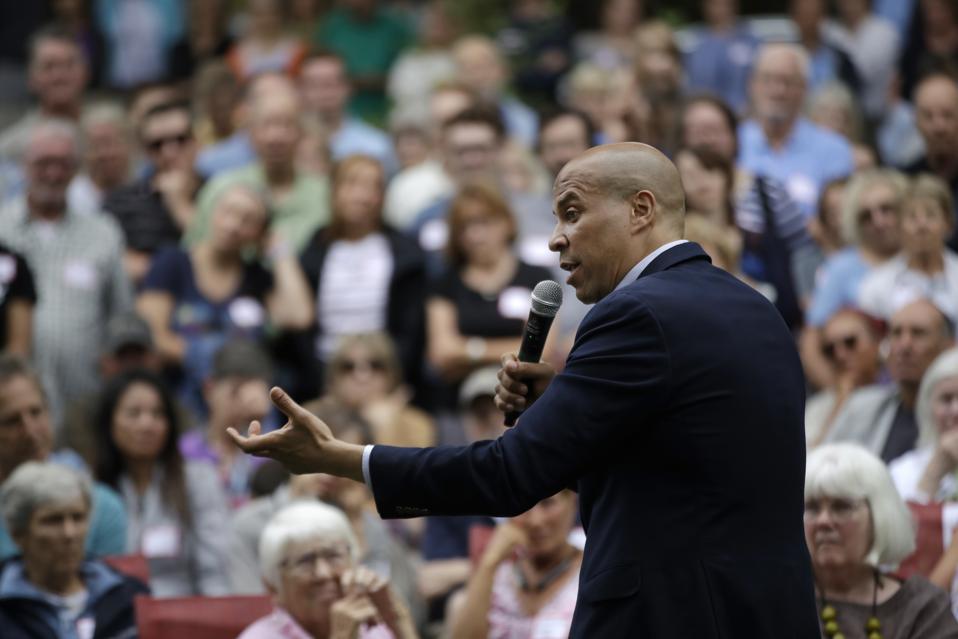
Democratic presidential candidate Sen. Cory Booker, D-N.J., speaks at a campaign event, Saturday, Aug. 17, 2019, in Portsmouth, N.H. (AP Photo/Elise Amendola)
ASSOCIATED PRESS
And with states such as Virginia, which according to Heather Crislip of Housing Opportunities Made Equal of VA, holding the top spot of three out of ten cities with the greatest number of evictions, further development in AI and algorithms as they pertaining to data searches, will only become more complex and invasive. "Landlords here seem to use eviction as a means of collection," snaps Crislip. "It's impacting people's lives in unnecessary ways."
But this issue is far from that of just affordable housing. Now with new proposed rule by Department of Housing and Urban Development (HUD) that is reported to end all possibility of accusing landlords of discrimination, living anywhere could get even trickier given how landlords could wield a deadly cocktail of algorithms to keep out those who don't meet their personal criteria.
As mentioned, landlords use tenant screening companies to apply algorithms to do credit and eviction checks, but now there are growing reports that some even use applicants’ social media accounts to track their leisure activities and create additional profiles of what they see to be an ideal tenant. In short, if you don't fit the algorithm, you could miss out on your dream location because, well, maybe you eat spend too much money going to gourmet juice bars. This would be considered discrimination, but good luck proving that or other levels in a world seemingly increasingly more hostile to just a normal scenario like securing housing.
According to an excellent recent piece in Slate on the new HUD proposal:
The Supreme Court held in 2015 that “disparate impact” discrimination—cases where there is no direct evidence of intentional discrimination, but still a disproportionate effect on the basis of protected classes like race, gender, or disability—is illegal under federal housing law. These cases, which rely on statistical proof of disparate impact rather than proof of differing treatment based on protected factors, have been a critical part of anti-discrimination law for decades.
The HUD proposal is designed to make it harder for plaintiffs to win these cases. For example, under the new proposal, instead of landlords having to defend a tenant screening policy on the grounds that it was the least discriminatory option, a prospective tenant would have to prove in court that it was not a valid choice in the first place. This is a major change for HUD, which in 2015 urged the Supreme Court to recognize the doctrine’s applicability
Such a move is massively important particularly due to the current racial climate in our country.
Evictions, credit issues, race, not a concern, you say? Not so fast.
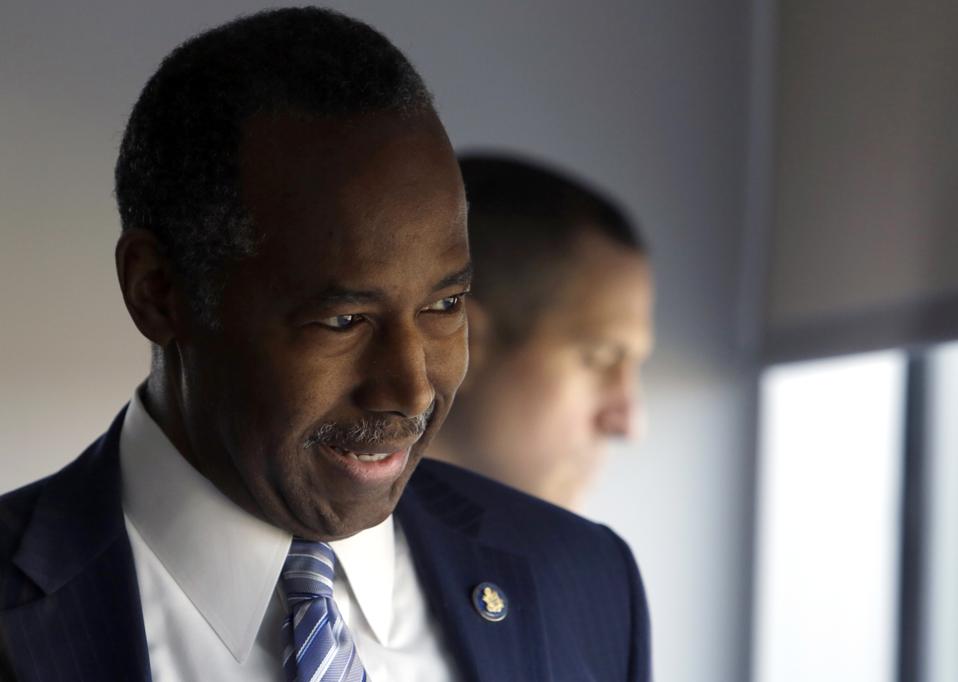
Housing and Urban Development Secretary Ben Carson is shown a view of Philadelphia by Lopa Kolluri, unseen, Chief Development and Operating Officer for the Philadelphia Housing Authority (PHA), Thursday Feb. 14, 2019, in Philadelphia. Carson was in
ASSOCIATED PRESSNewer, unyielding algorithms can even impact you if you’re planning to move to a new rental or apply for a mortgage even if you pay your bills on time. Indeed the latest trend is to be, penalized for good behavior and have it decrease your credit score. I know. It happened to me.
I had a retail card issued by Comenity bank. This bank, which seemingly has a near-monopoly on retail cards, conducts periodic checks on its cardholders' credit. If the algorithm sees something it does not like, in my case, a new card I accepted in order to get the miles and paying it and others all on time, my credit limit was drastically cut by Comenity, and it sent my credit score into a tailspin. Again, for paying on time based on what the algorithm thought could happen in the future.
When I asked how this could possibly happen, I received the standard response via email from a representative at Comenity Bank:
Through our Comenity banking operations, we provide market-leading private label, co-brand and commercial credit card programs for more than 145 brands and 41 million active credit cardmembers, leveraging extensive marketing and loyalty expertise to provide payment offerings that meet the needs and preferences of our brand partners’ valued cardmembers
Given the significant complexities and proprietary nature of Comenity’s underwriting processes and lending practices, we cannot disclose specific details related to them. However, for related information, you may find portions of Alliance Data’s most recent Sustainability Report helpful under our Fair and Responsible Banking section.
Which, essentially, means nothing to a future landlord's algorithm as it talks to FICO's algorithm and simply sees a number.
Truly in an era of algorithms powered by deepening AI, the fundamental right of housing seems a trickier labyrinth each day. "Credit score is an extremely important indicator in terms of the American economy, which has one of the most credit driven societies in the world," explains Peter Gloger, Senior Machine Learning Engineer at Netguru.
He continues, "The models used in assessing credit risk are based on linear relationships, that is, logistic regression. Let's assume the model determining it used the variable ‘client uses other credit cards.' In this case, the answer would turn from ‘No’ into a ‘Yes’ just like that. We can also assume the data the model was trained on had such examples and in most cases getting another card meant problems with repaying the loan for the original card. But that may not be the case in reality. At any rate, yes, this reduction of credit limits starts a chain reaction of reducing the FICO score."
Gloger says that initially, machine learning algorithms were able to capture the complex interconnections between credit score indicators much more precisely than the more traditional methods but that they had one very important drawback. Most of them lacked intuitive explainability essential for the customers and regulators to trust in their judgment. "Currently, a lot of effort is being put into creating modern methods in a way that clearly gives insights on how the algorithm made its decision without compromising its accuracy and complexity. The sooner this gets adopted the better, for both banking institutions and their customers," explains Gloger.
However, Kareem Saleh, an EVP at ZestFinance, an AI software company for credit, and one who spent several years with the Obama Administration gives even deeper insight. "The people who make traditional credit scores are not trying to be biased against anyone, and they strive to be fair. But a seemingly objective model trained on historical data is going to reflect and exacerbate historic discrimination and bias."
For example, he says that the fact that certain groups have not historically had ready access to safe and responsible credit could incorrectly predict that people in those groups high credit risks. It can be hard to break into the traditional credit system if you have historically been excluded. Indeed, even length of credit history is 15% of a traditional credit score and such reliance on history obviously penalizes Millennials who are apartment and house hunting, too.
Saleh explains, "Machine learning (ML) expands the amount of data you can take in, uses different kinds of math, and reduces the dominance of any one signal that goes into a traditional credit score. ML can find good credit signals in other places such as transaction data and customer relationship data. For these reasons, it can increase access to historically disadvantaged groups. An auto lender we worked with increased its approval rates among Millennials by fivefold with no added risk."
But the underbelly of these algorithms might be the most interesting. Saleh says that, of course, by law banks cannot use race or another protected characteristic directly as an input into a credit model. That said, banks do collect race, sex, and national origin information for some products in order to conduct fair lending analysis. When they don’t collect that information directly, they use a methodology called BISG to make estimates about protected class status by looking at a borrower's last name and zip code.
"This discriminatory bias can be injected in other ways. The variables used in a model might be acting as proxies for race or another protected characteristic. For example, where someone lives or their behavioral patterns, like whether they subscribe to Ebony magazine, might function as a proxy for race, even if race is not directly used as a variable."
He adds, "Things get even more complicated for AI/ML models which, if they’re done incorrectly, can function as a 'black box.' Without visibility into how seemingly fair signals interact in an AI/ML model to hide bias, lenders will make continue to make decisions which tend to adversely affect borrowers."
Saleh notes that there is, naturally, increasing public and congressional awareness of these problems. However, he adds, "AI/ML has the potential to asses hundreds of data points, rather than a few dozen, to determine if a handful of better credit signals elsewhere in a consumer’s profile could counterweight the fact that they've opened up a new card account."
Taras Kloba Head of Big Data Center of Excellence at Intellias concurs, “Artificial intelligence is one of the most promising technologies with the potential to revamp the financial sector today. AI platforms allow banks to automate processes, better understand customers, and advance overall service quality."
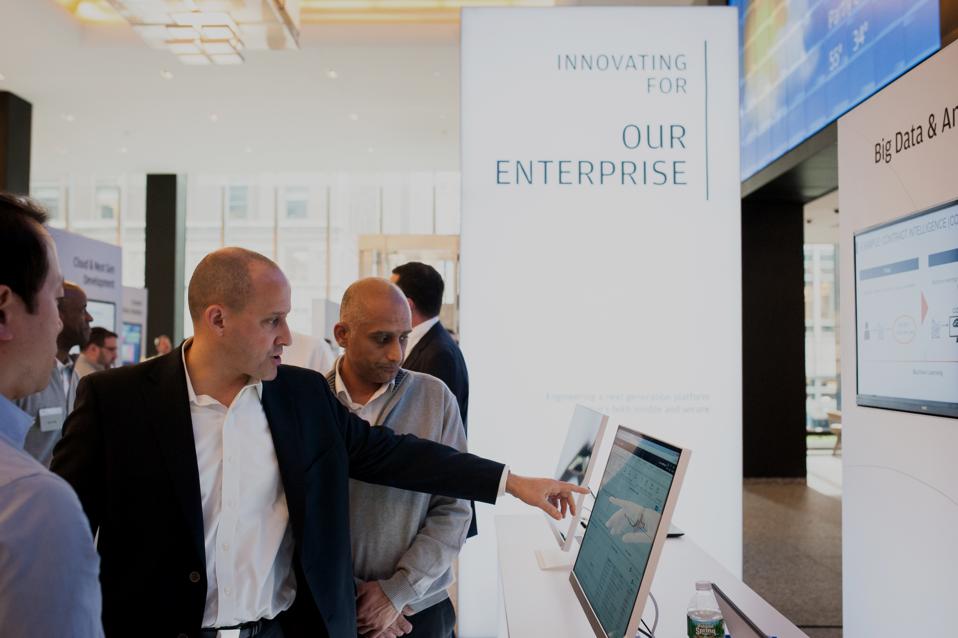
Matt Zames, chief operating officer of JPMorgan Chase & Co., center, views JPMorgan & Chase Co. Markets software kiosk on the eve of the company's annual Investor Day in New York, U.S., on Monday, Feb. 27, 2017. At JPMorgan Chase & Co., a
© 2017 BLOOMBERG FINANCE LPBut somehow the fact of the matter is, currently, it is absolutely not acting as an aide to most of us.
And if you're thinking of getting out of the credit, algorithm, machine learning maze altogether as you prepare to move and simply cancel a few credit cards, think again. Exiting this network may be harder than leaving the mafia in the old days. "It might seem logical to close cards to stop any problems from happening," says Gloger. "But keep in mind that banks use an algorithmic parameter called a 'Credit Utilisation Ratio' which might change your score drastically when you close a credit card. This too can impact your score and sway those you need to persuade for housing."
Clearly the area is becoming more complex and deserves greater discussion immediately. Where we live can often make getting to next rung in life easier or harder for ourselves and/or our families. Our residences and neighborhoods help to create peace of mind, provide access to education, even oftentimes determine the quality of groceries you can readily access. Those in control are playing a dangerous game because after all, it's hard to reason with a computer once it has made a final decision.
In addition, be aware. There is a 60-day period beginning August 19 when public comments are accepted on the new HUD proposal mentioned above. Additional information to do that can be accessed here.